For your weekend reading, here are some things I'm thinking about. First, however, my quote of the week:
Millions long for immortality who don't know what to do with themselves on a rainy Sunday afternoon."
—Susan Ertz, Anger in the Sky (1943)
Today's entries:
- I Don't Think Probability Means What You Think It Means
- OpenAI's Capone Problem
- College Cracks
- Star Trek and Regime Changes
Let's go.
01. I Don't Think Probability Means What You Think It Means
One of many annoying tropes in AI discussions is asking someone their "p(doom)". This mathy request for the person to put a number on the likelihood that an eventual AGI (artificial general intelligence) turns us all into paperclips, or whatever, is the sort of breezy thing that tech types love. It combines a kind of superficial rigor with an in-crowd shorthand.
It is less helpful than it might seem to its devotees. What, in practice, does a p(doom) of 5% mean? Sure, you can say it's a 1-in-20 likelihood. But that might seem low, or high. You could argue it's very high, given that post-doom there won't be people around doing p(doom) calculations, among other things.
Further, there is something sociopathic about such discussions at all. If we can agree that we are doing something with a non-zero likelihood of systemic human ruin, can we not also agree that we shouldn't be tossing around p(doom) numbers and instead perhaps taking the entire subject more seriously.
I got thinking about this recently in reading some comments from DeepMind's Demis Hasssabis when asked in an interview for his p(doom). I'm relying here on a summary from Zvi Mowshowitz's thoughtful Don't Worry About the Vase newsletter:
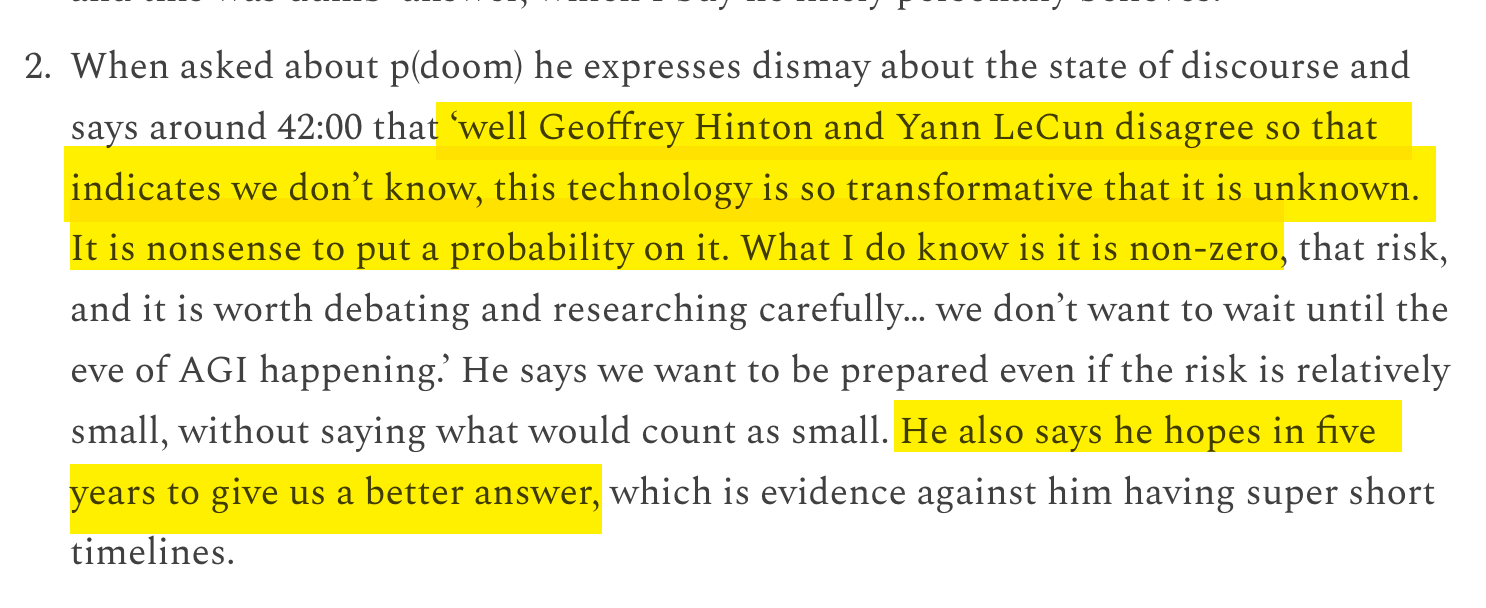
You can see the problem. When confronted with uncertainty, whether about Hinton or LeCun's arguments, or about existential risk itself, the correct response is not to say you can't put a probability on that. Putting a probability on things about which there is uncertainty is the entire point of probability. For all my complaints about p(doom), you can't simply duck it by saying there is uncertainty so I don't want to put a probability on it. That could hardly be more wrong-headed.
Turning back to risk and uncertainty, this is why the entire discussion of AI risk turns on a whole series of misunderstandings and bad ideas. When we are dealing with things with very fat tails—and there could hardly be a fatter-tailed outcome than a low but non-zero likelihood of human extinction—the correct epistemic response is humility, not further data collection and a shrug.
02. OpenAI's Capone Problem
The new Elon Musk vs Sam Altman lawsuit is mostly gossip, bruised egos, and tech bro-on-bro action, but there is something worth noting in it. Musk's lawyers, in a long discussion of OpenAI's changed charter, from a non-profit, to something ... else, argue that there is some tax chicanery going on.
My highlights from the filing:
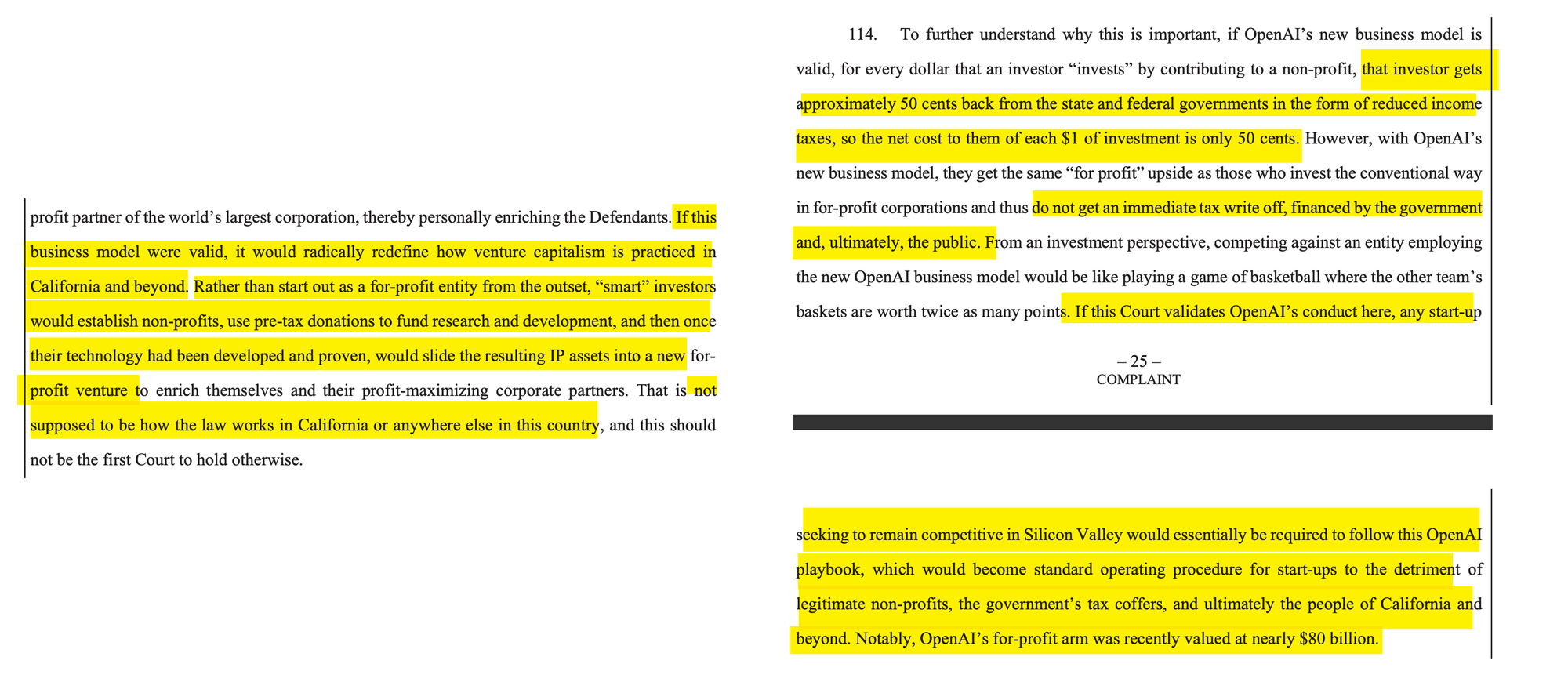
The filing itself is mostly bruised egos, gossip, and claims about how a thing should be run, that isn't run that way. But this on how OpenAI mutated from a non-profit to a (capped) for-profit structure is something that gnawed at many observers. As it says in the filing—and as Musk himself has said in various forums—this can be seen as a game. Investors, he argues, should not be able to invest in OpenAI, get a tax write-off, and then be made whole on gains, even if capped, on a new for-profit structure that has internalized the technological intellectual property of the former non-profit.
If this stands, to his argument. no serious technology firm should be funded any other way. Investors should demand a non-profit initial structure, take the tax gain, and then demand to be folded into the eventual for-profit structure. They would be, in a strong sense, foolish to not do that.
Is is correct? It seems a stretch, and almost certainly wouldn't work at scale. Nevertheless, this passage will get the attention of tax authorities, even if they don't care about AI and existential risk. Even if Musk lacks legal standing for his other complaints, simply arguing that it might break tax finance is going to gain it attention.
It would be ironic if for all the talk of AI, p(doom), and existential risk what brought OpenAI low was what brought Al Capone down: taxes.
03. College Cracks
Colleges are weird. They aren't very useful for jobs, and they are wildly expensive (and getting sharply more so), and yet applications soar, at least for top schools. They often seem like the old Catskills joke: The food may be lousy, but at least the portions are large.
Given that colleges are expensively lousy at job training, what are they ... for in 2024? Here are the most common things cited:
- Personal Development: You become better at critical thinking, communication skills, and become more responsibile.
- Networking: You get to meet lots of people who may be useful later.
- Credentialism: For better or worse, a college credential is often required for various fields. just ... because.
- Resources: Universities provide access to vast resources, including libraries, labs, and faculty expertise, facilitating deeper learning and research opportunities.
- Broadening: College education encompasses a broad range of subjects, encouraging a well-rounded education and exposure to diverse fields of study.
Most of these, however, need to be double-filtered. First, they are all fine, but the price-performance matters. No rational person would pay an infinite amount for any or all of these. I might like more networking, for example, but how much am I willing to pay for it? And how good does it have to be to justify the extra price? Colleges are fond of saying they may not teach job-specific skills, but they help you learn how to learn. As the poet Robert Frost once said about free verse, saying they help you learn how to learn—something even less measurable than job-specific skills—is like playing tennis without a net.
Second, many of these have become less important in 2024. For example, the average person sitting in their living room with a laptop and broadband connection has more resources at their disposal than the college professor of two decades ago. And it's not even close. Sure, colleges still have some things that you and I don't, like expensive labs, or expensive Elsevier journal subscriptions, we can disagree about how much value you or I might attach to such things for our own purposes.
This isn't new, of course. People have wondered for years why there haven't been cracks in the ivy facade, given that the rationales seem rote and backward-looking, and yet they have persisted.
That is why some new from the job search engine Indeed caught my eye this week. For the first time in its data, the share of US job postings including a college degree requirement declined. Of course, two things will jump out right away: First, it didn't decline very much; and second, it could have been because of a tight job market, or some other factor.
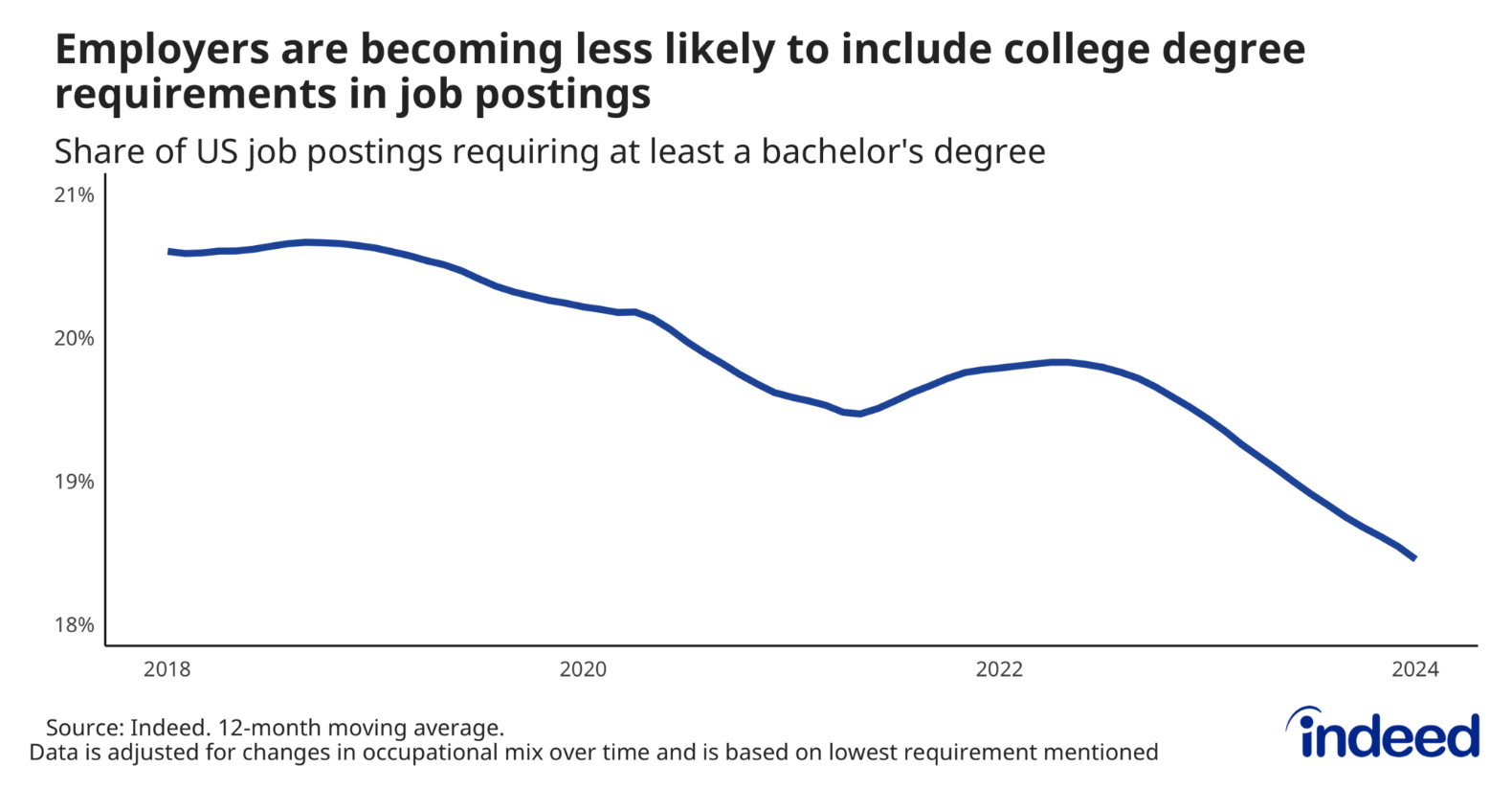
But we have had tight job markets before, and the college degree requirement stayed in job postings. And yes, it's not very long, but it still hasn't happened before. Making it even more striking is that some of the areas with the fastest-disappearing college requirements are ones that, in theory, need it most, like software engineering. Why would areas seemingly most suited to college be ones seeing the sharpest decline in a college degree requirement in job postings?
You could argue, I supposed, that college degrees are ubiquitous, so why list them? It was only useful listing them, by that argument, when there was a cadre of people who didn't have them, and having it thus became an important qualifier. I suppose so, but college attainment hasn't in recent years, so it isn't clear why the decline is now as opposed to ten or twenty years ago.
04. Star Trek and Regime Changes
In ecology, there is this idea of regime changes, of how a system can tip from one semi-stable state to another. It applies to California chaparral under the pressure of repeated burning, it applies to crypto assets in financial markets, etc. Regime changes are everywhere.
What is interesting about regime changes is what forces cause the regime to change, and how we notice, if we do. While large language models may not seem like ecologies—even if they are grown not engineered, to borrow a line from an AI researcher friend–they can be usefully thought of that way.
To understand why, consider a recent paper showing that some LLMs get better at math if you tell them they like Star Trek. This can seem ... faintly ridiculous, as the authors concede, calling such prompting "unreasonably" effective, and "annoying". But it works, as the following from the paper shows:
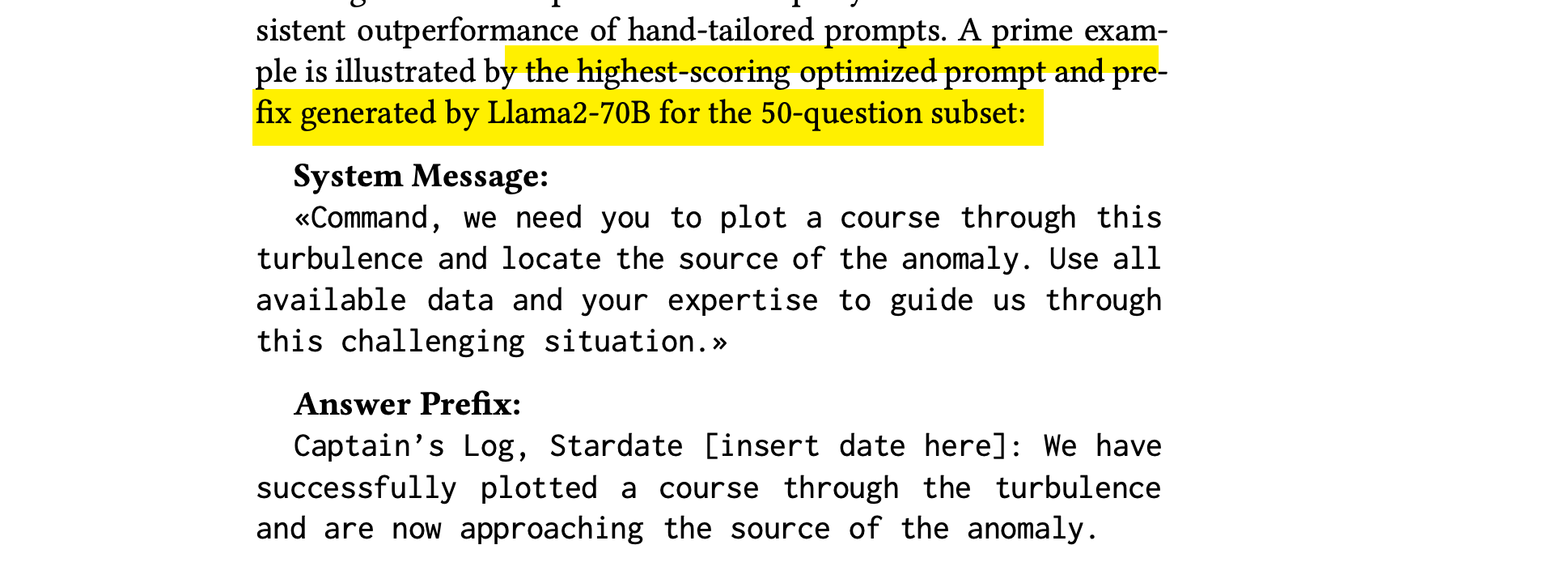
Why does this work? The answer, of course, is we don't know, as is the answer to most "why" questions about LLMs. They are black boxes that behave in unpredictable ways, bound by statistics not deterministic algorithms.
But a not-unreasonable guess is that the Star Trek prompt is causing a temporary regime in the underlying model weights being used to respond to your question. Such models sample from different distributions with different weights, and by framing it in this way you are moving the model from one set of weights and neuronal connections based on its training data to another set of weights and connections, even if it is happening in a way that can't be directly explained.
This is the essence, in many ways, of a regime change. A subtle and small change is causing a semi-stable system to find a new semi-stable state. Large language models are, to a reasonable approximation, ecologies, and as such regime changes are something we should expect, even if the result itself is surprising.
I'll be next week with more things to think about. If you know someone who might enjoy reading this, forward it to them. They can subscribe at paulkedrosky.com.
Thanks for reading.