For years, the Vermont town of Pomfret has been a destination for people wanting to see fall scenery. But this year the crowds of tourists have exploded, in large part because of a surge of influencers in pursuit of the perfect autumnal shot for their Instagram and TikTok followers. And then their followers follow them, and it builds and builds.
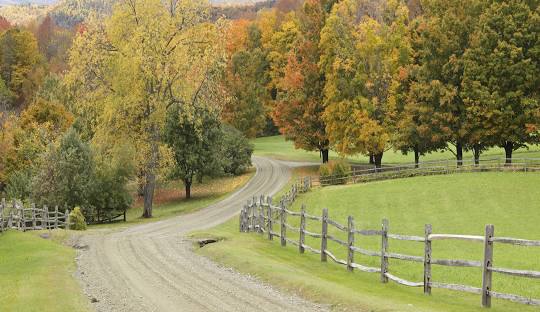
While this might seem merely wearying and cliched, it is much more than that for residents. Narrow roads are jammed with hundreds of cars, drones are buzzing overhead, and influencers have even brought outhouses and portable change rooms as they stalk the perfect shot. The town finally closed a road to manage the influx.
The following paired pictures from a story on the fall foliage frenzy in Pomfret drive the point home, with the picture on the left from in front of a lens, and the one on the left taken behind the scenes.
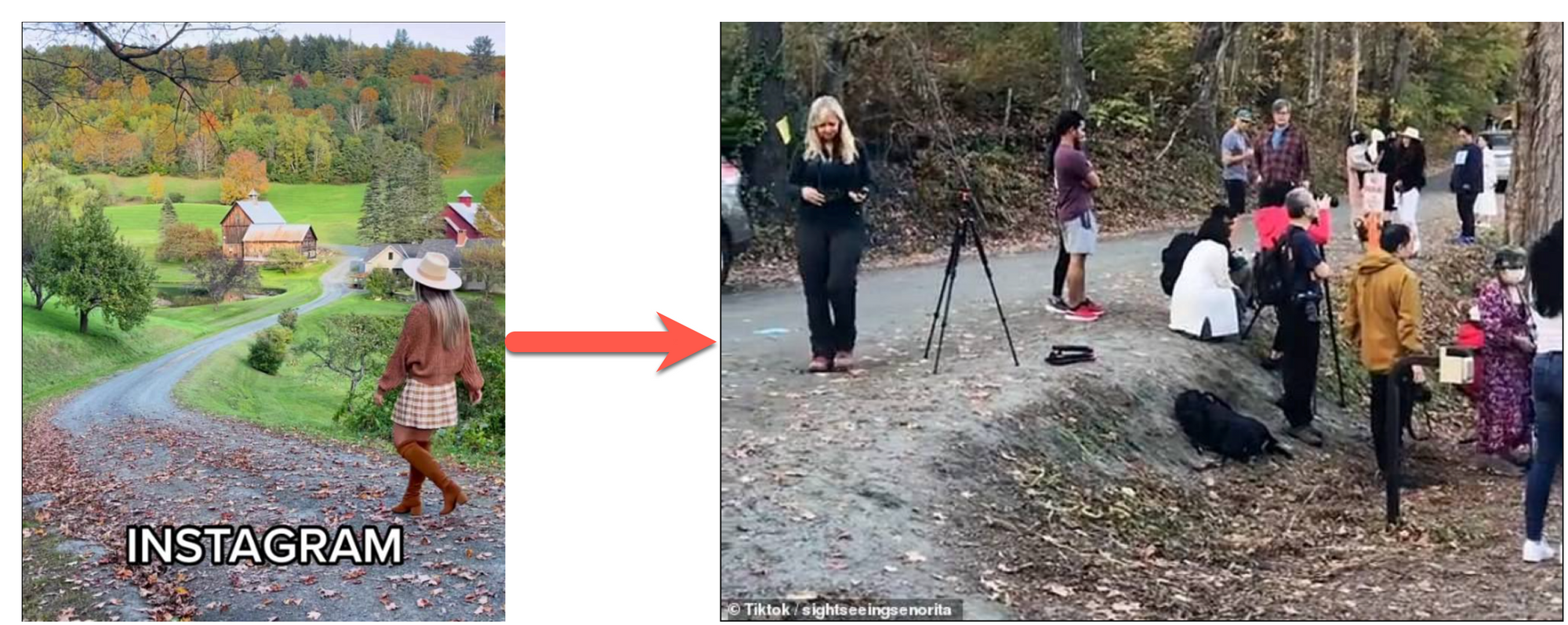
This is an increasingly common problem. Certain sites at national parks are overwhelmed, while other parks see less traffic than in decades. Some destinations see so much traffic that the experience becomes catastrophic for the thing itself, but the crowds keep coming. When a location or trend becomes the subject of mimicry cascades, it can lead to unforeseen consequences, sometimes overwhelming systems (or towns) unprepared for such rapid popularity.
In the balance of this post, I use a Monte Carlo simulation-based agent system to show how mimicry emerges, flowers, and spreads. As the fall foliage crowds in Pomfret illustrates, its implications are very much grounded in reality.
Mimicry and Sync in Agent-Based Systems
The spontaneous emergence of sync in systems is important. For example, it has been demonstrated in nine species of fireflies that live in the Great Smoky Mountains National Park. These fireflies are known to synchronize their flashing light patterns, and flashing synchrony emerges at the individual level, not via some top-down structure.
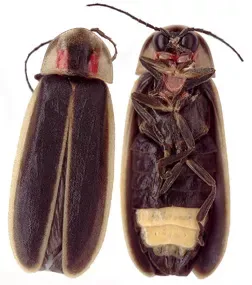
Similar phenomena can be observed in heart muscles, where oxygen-depleted blood enters through the top right chamber of the heart. That blood is then pumped into the right ventricle and into the lungs via the pulmonary artery, where it absorbs oxygen. This oxygen-rich blood is then pumped into the top-left chamber of the heart, and then, eventually out into the body through the aorta. All four chambers must be in sync, working together. If this ceases the condition is called ventricular dyssynchrony,, and it is very serious, leading to death if untreated. Sync has serious consequences.
Studying these networks is a rich area of work. One way to do so is via agent-based systems: powerful tools for simulating the interactions and behaviors of individual agents within a given environment. They allow us to model complex systems, such as market dynamics, ecological systems, or even social networks, by understanding how individual actors influence and react to one another. One intriguing phenomenon that can emerge from these systems is mimicry—the tendency of agents to replicate the behaviors or characteristics of other agents.
In a recent exploration, I simulated an agent-based system that featured two types of agents: "normals" and "influencers". I wanted to see under which circumstances mimicry would arise, and how rapidly it would spread throughout the agent population. The simulation results were surprising and insightful.
Setup and Methodology
I set up a population of 1,000 agents, where the majority were "normals" and a minority were "influencers". We tested three different scenarios: one with no influencers, one with 1% influencers, and one with 10% influencers. The key difference between normals and influencers is that the former are aware of and likely to imitate the latter, but the reverse is not true.
Agents began the simulation unaware of each other. In each turn, the model evaluated two things:
- Whether an agent becomes "aware" of another agent (whether a normal or an influencer) based on different predetermined likelihoods.
- If an agent becomes aware of another agent, whether they start mimicking that agent.
Different interaction probabilities were set based on the type of agent interaction. For instance, "normals" had a much higher likelihood of becoming aware of "influencers" than of other "normals". Similarly, upon awareness, a "normal" agent was much more likely to mimic an "influencer" than another "normal".
The model ran until 80% of the population was mimicking one another, or 1,000 turns passed without convergence to the 80% hurdle.
Findings
The impact of influencers was striking. In the scenario with no influencers (0%), it took a substantial 653 turns for 80% of the agents to mimic each other. However, with just a 1% influencer population, the number of turns required dropped by 62% to 243. And with 10% influencers? A mere 19 turns were required to reach the 80% mimicry threshold.
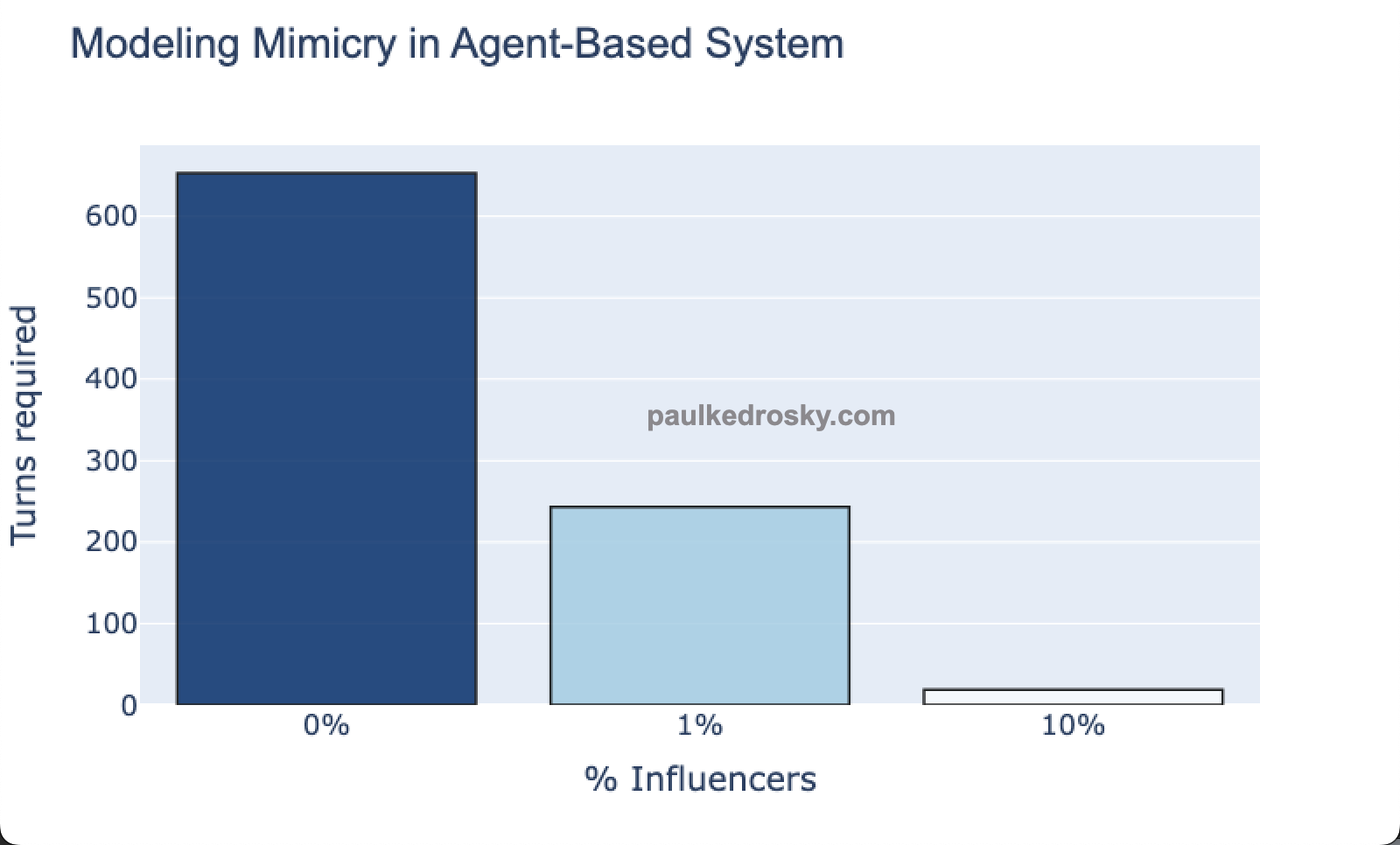
The upshot? Even a small percentage of influencers—widely imitated and highly connected nodes—can significantly accelerate the spread of mimicry within a system. It also demonstrates how certain agents, based on their attributes or positions in a network, can exert disproportionate influence over the system's overall behavior.
Understanding the Implications
The power of mimicry in agent-based systems is reminiscent of real-world phenomena we observe in various fields:
- Marketing and Social Media: Brands often collaborate with influencers, knowing that their reach and impact can drive trends and purchasing decisions among the broader population. We see this all the time, even if we aren't aware of the games being played in the background.
- Financial Markets: When influential investors make moves, others often mimic them, leading to market shifts.
- Cultural Trends: Prominent figures in culture, from musicians to politicians, can introduce new words, ideas, or behaviors that quickly become mainstream.
All of this has become more important in recent years. While mimicry has always existed, it was never as fast, as prone to narrow convergence, nor so easily manipulated. Through a combination of social media, imitative behaviors, and related peer effects, point masses of humanity can descend on anything with no notice, from financial markets, to a single Vermont road with fall foliage.
Insidiously, as shown in the simulation, the addition of a small number of influencers—widely followed and imitated individuals or groups—drastically reduced the time required for the majority of the agent population to engage in mimicry. Realizing this, in the case of Pomfret, the town has pleaded with influencers to stop coming, and stop sharing photographs of the town's leaves.
Concluding Thoughts
Agent-based modeling offers a lens into the intricate dance of individual components within a system. The unexpected emergence of mimicry, especially under the influence of a small subset of agents, underscores the interconnectedness and complexity inherent in such systems. We see this behavior across biology, financial markets, and social networks, leading to new sources of instability, crowding, and fragility.
Many such systems are highly non-linear, where a small input change (like the addition of a few influencers) can lead to disproportionately large output changes. In case the case of Pomfret, it can cause more people to descend on a physical location than the location can handle. And the crowds become their own confirmation, as mimicry begets mimicry, the large numbers of people doing the same things provides perversely validating evidence.
Talking to Papers
In the second part of this note I use an LLM to talk to a new paper ("Mutilation of the tree of life via mass extinction of animal genera") co-authored by Stanford ecologist Paul Ehrlich.